SYMPOSIUM 11: Impact of exposure measurement error (ME) on the health effect estimates: quantification and correction
Tracks
Skalkotas Hall
Tuesday, September 20, 2022 |
11:45 AM - 1:26 PM |
Skalkotas Hall |
Overview
Skalkotas Hall
Speaker
Prof. Klea Katsouyanni
Professor
National And Kapodistrian University Of Athens And Imperial College London
O-SY-049 Impact of exposure measurement error (ME) on the health effect estimates: introduction
Abstract
Background/Aim: Although it is widely accepted that air pollution exposure, at least to pollutants of ambient origin, has adverse health effects, the quantitative effect estimates incorporate considerable uncertainty, especially because the relevant exposure (namely individual or population exposure to pollutants of ambient origin) is so difficult to measure accurately. The resulting exposure Measurement Error (ME) is a neglected but potentially important problem resulting in bias and loss of accuracy in health effect estimates. This Symposium will focus on recently published results on the bias in effect estimates due to exposure measurement error.
Methods: Measurements of air pollutants concentrations from fixed site monitors or ad-hoc campaigns have been used in epidemiological studies assessing the associations between exposure to pollutants and multiple health outcomes, and also models (statistical, dispersion or hybrid) have been extensively developed and applied. The magnitude and direction of bias resulting from ME in exposure estimation has mainly been evaluated in simulation or validation studies.
Results: Recent publications show that bias from ME of commonly applied exposure models is mainly towards the null and can be substantial. Only under specific and relatively rarely observed values of correlations and variance ratios upward bias is observed. Other studies which use more sophisticated “hybrid” models show smaller bias.
Conclusion: In this Symposium we will attempt to quantify the importance of exposure ME in the valid estimation of health effects of air pollutants. We will present results from recent studies which quantified the impact of various types of exposure measurement error on the effect estimates for health outcomes and evaluated correction methods.
Key words: exposure measurement error; air pollution; bias; effect estimates; correction methods
Methods: Measurements of air pollutants concentrations from fixed site monitors or ad-hoc campaigns have been used in epidemiological studies assessing the associations between exposure to pollutants and multiple health outcomes, and also models (statistical, dispersion or hybrid) have been extensively developed and applied. The magnitude and direction of bias resulting from ME in exposure estimation has mainly been evaluated in simulation or validation studies.
Results: Recent publications show that bias from ME of commonly applied exposure models is mainly towards the null and can be substantial. Only under specific and relatively rarely observed values of correlations and variance ratios upward bias is observed. Other studies which use more sophisticated “hybrid” models show smaller bias.
Conclusion: In this Symposium we will attempt to quantify the importance of exposure ME in the valid estimation of health effects of air pollutants. We will present results from recent studies which quantified the impact of various types of exposure measurement error on the effect estimates for health outcomes and evaluated correction methods.
Key words: exposure measurement error; air pollution; bias; effect estimates; correction methods
Dr. Dimitris Evangelopoulos
Research Fellow
Imperial College London
O-SY-049a Identifying determinants and characterizing the type of exposure measurement error in association with different error-prone exposure assessment methods
Abstract
Background/Aim
In air pollution epidemiology, error-prone exposures are used to investigate the health effects of exposure to outdoor pollution. This leads to measurement error (ME) bias of varying size and direction. We seek to quantify ME magnitude, type (classical/Berkson/mixture), and determinants, to be able to use the most appropriate methods for error correction. Personal exposure to pollution from ambient origin has been defined as the true exposure and ME as the difference between the latter and any surrogate exposure.
Methods
Within the MELONS project, we collected personal data from multiple monitoring campaigns in London for PM₂.₅, NO₂, O₃ and BC. We compared those with measurements from the monitoring network, modelled concentrations at fine spatial scales or methods incorporating indirect adjustment of these estimates for the time-activity patterns in the postcode sector of the participants. Individual- and area-level characteristics, e.g. age, sex, socioeconomic status, temperature, were assessed as potential ME determinants.
Results
Preliminary analysis using long-term exposure estimates has shown that personal exposure from outdoor sources to PM₂.₅ and NO₂ (Mean (SD): 6.4 (3.0) μg/m³ and 7.1 (4.2) μg/m³ respectively) was largely overestimated when surrogate exposures were used (up to 9.9 (2.2) μg/m³ and 39.5 (11.0) μg/m³ respectively). Indirect time-activity adjustment for the nearest monitor estimates reduced ME magnitude and variability substantially. The types of error also varied by the surrogate used. Age and proximity to the nearest monitor were found to drive ME. Day-to-day ME variation and potential time-varying error determinants will also be examined.
Conclusions
The use of surrogate exposures for the investigation of the health effects of long-term exposure to outdoor air pollution can distort the epidemiological estimates. Information about the ME structures and its determinants can be used for correction and the identification of the true exposure-response functions.
Keywords
Air pollution, Measurement error, Mixture error, Error determinants
In air pollution epidemiology, error-prone exposures are used to investigate the health effects of exposure to outdoor pollution. This leads to measurement error (ME) bias of varying size and direction. We seek to quantify ME magnitude, type (classical/Berkson/mixture), and determinants, to be able to use the most appropriate methods for error correction. Personal exposure to pollution from ambient origin has been defined as the true exposure and ME as the difference between the latter and any surrogate exposure.
Methods
Within the MELONS project, we collected personal data from multiple monitoring campaigns in London for PM₂.₅, NO₂, O₃ and BC. We compared those with measurements from the monitoring network, modelled concentrations at fine spatial scales or methods incorporating indirect adjustment of these estimates for the time-activity patterns in the postcode sector of the participants. Individual- and area-level characteristics, e.g. age, sex, socioeconomic status, temperature, were assessed as potential ME determinants.
Results
Preliminary analysis using long-term exposure estimates has shown that personal exposure from outdoor sources to PM₂.₅ and NO₂ (Mean (SD): 6.4 (3.0) μg/m³ and 7.1 (4.2) μg/m³ respectively) was largely overestimated when surrogate exposures were used (up to 9.9 (2.2) μg/m³ and 39.5 (11.0) μg/m³ respectively). Indirect time-activity adjustment for the nearest monitor estimates reduced ME magnitude and variability substantially. The types of error also varied by the surrogate used. Age and proximity to the nearest monitor were found to drive ME. Day-to-day ME variation and potential time-varying error determinants will also be examined.
Conclusions
The use of surrogate exposures for the investigation of the health effects of long-term exposure to outdoor air pollution can distort the epidemiological estimates. Information about the ME structures and its determinants can be used for correction and the identification of the true exposure-response functions.
Keywords
Air pollution, Measurement error, Mixture error, Error determinants
Dr. Joel Schwartz
Professor
Harvard School of Public Health
O-SY-050 Accounting for space and time in measurement error corrections
Abstract
Measurement error is endemic in epidemiology and arguments over the direction of bias persist. Modern high-accuracy models of ambient concentrations of air pollutants have reduce error. We illustrate the use of machine learning algorithms to estimate the spatial and temporal variation of exposure error in simulation studies to estimate the direction and extent of bias in air pollution epidemiology using linear, nonlinear, and threshold relationships. We also illustrate the use of regression calibration models that account for differences in calibration across space. We also discuss the parallel between using ambient instead of personal exposure and intention to treat analyses.
Ms. Barbara Nicholas (nee Butland)
Senior Lecturer In Medical Statistics
St George's, University Of London
O-SY-051 Using SIMEX and regression calibration to correct for mixtures of classical and Berkson measurement error
Abstract
BACKGROUND AND AIM
Measurement error in modelled air pollution data is complex consisting of classical and Berkson error components. As part of the MELONS project, we investigated the adequacy of regression calibration and SIMEX in correcting health effect estimates when measurement error contains both components and may also be spatially correlated.
METHODS
In a simulation study of 5,000 subjects, we considered scenarios based on the amount of total measurement error (either 20% or 40% of the variance in the true annual average pollutant concentrations); the proportion of error that was classical (0%,20%,40%,60%, 80% or 100%); and whether total measurement error was homoscedastic or heteroscedastic. Within each simulation, we obtained a dataset of true, and error prone annual average pollutant concentrations and outcome data linked to the true by a hazard ratio of 1.041 per 10µg/m³. The error prone pollutant concentrations were then used as the exposure variable in a Cox regression, and for error correction, a 5% hypothetical validation sub-sample of the data were randomly selected and used to estimate the classical and Berkson error variances. Based on 1,600 simulations per scenario we investigated mean percent bias in the estimated log hazard ratio and the ability of regression calibration and SIMEX to correct for this bias.
RESULTS
Mean percent bias in the uncorrected log hazard ratio was typically negative (i.e., towards the null) ranging from -27.4% to +2.0% across scenarios. Mean percent bias in the corrected estimates ranged from -4.0% to +5.7% for regression calibration and -6.0% to +4.2% for SIMEX.
CONCLUSIONS
Across a range of scenarios varying the mix of classical and Berkson error and allowing total measurement error to vary spatially, SIMEX and regression calibration produced corrected log hazard ratios with relatively small mean percent bias.
KEYWORDS
Measurement Error, SIMEX, Regression Calibration
Measurement error in modelled air pollution data is complex consisting of classical and Berkson error components. As part of the MELONS project, we investigated the adequacy of regression calibration and SIMEX in correcting health effect estimates when measurement error contains both components and may also be spatially correlated.
METHODS
In a simulation study of 5,000 subjects, we considered scenarios based on the amount of total measurement error (either 20% or 40% of the variance in the true annual average pollutant concentrations); the proportion of error that was classical (0%,20%,40%,60%, 80% or 100%); and whether total measurement error was homoscedastic or heteroscedastic. Within each simulation, we obtained a dataset of true, and error prone annual average pollutant concentrations and outcome data linked to the true by a hazard ratio of 1.041 per 10µg/m³. The error prone pollutant concentrations were then used as the exposure variable in a Cox regression, and for error correction, a 5% hypothetical validation sub-sample of the data were randomly selected and used to estimate the classical and Berkson error variances. Based on 1,600 simulations per scenario we investigated mean percent bias in the estimated log hazard ratio and the ability of regression calibration and SIMEX to correct for this bias.
RESULTS
Mean percent bias in the uncorrected log hazard ratio was typically negative (i.e., towards the null) ranging from -27.4% to +2.0% across scenarios. Mean percent bias in the corrected estimates ranged from -4.0% to +5.7% for regression calibration and -6.0% to +4.2% for SIMEX.
CONCLUSIONS
Across a range of scenarios varying the mix of classical and Berkson error and allowing total measurement error to vary spatially, SIMEX and regression calibration produced corrected log hazard ratios with relatively small mean percent bias.
KEYWORDS
Measurement Error, SIMEX, Regression Calibration
Prof. Donna Spiegelman
Director, Center For Methods In Implementation And Prevention Science (cmips), Yale School Of Public Health
Yale University
O-SY-052 Empirical measurement error bias correction for the effects of functions of the exposure history: cumulative average PM2.5 in relation to all-cause mortality
Abstract
Cohort studies in air pollution epidemiology construct the exposure metric as a cumulative average over a pre-determined time window. We present valid methods for empirically estimating the time window, and simultaneously estimating the exposure effect from this complex variable that is a function of multiple exposure measurements over time, correcting for exposure measurement error bias using validation data. Air pollution validation studies will be briefly presented. Then, methods will be illustrated in the Nurses’ Health Study’s investigation of PM2.5 on all-cause mortality. Valid confidence interval construction will be considered, and publicly available software for conducting these analyses will be shown.
Chair
Dimitris Evangelopoulos
Research Fellow
Imperial College London
Klea Katsouyanni
Professor
National And Kapodistrian University Of Athens And Imperial College London
Technical Support
Chair
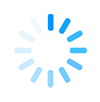