INVITED SESSION 1: Causal Inference and Machine Learning
Tracks
PLENARY HALL (Friends of Music)
Monday, July 22, 2024 |
11:00 AM - 12:33 PM |
PLENARY HALL (M1 building upper floor) |
Details
Machine learning and causal inference have both surged over the past decades, each carrying their own promise of superior data analysis. More recently, they were merged to bring stronger evidence. Double robust causal inference has become a vehicle that motivates joining causal inference and machine learning. It is however often ill understood. In a first talk Erin Gabriel (Copenhagen University) will revisit the concept and practice of double robustness, cautioning against the common misbelief that any combination of IPW with outcome regression yields double robustness. In a second talk, Martin Spindler (University of Hamburg, co-author of the double ML package in R, introduces the new field of causal machine learning for causal inference in high dimensional data settings. In a final talk, we hear from Maya Peterson (Berkeley Center for Targeted Machine Learning and Causal Inference) about machine learning for sequential multiple assignment randomized trial analysis to support precision medicine.
Presentations
Prof. Erin Gabriel
Associate Professor
University of Copenhagen, Denmark
Prof. Martin Spindler
Professor
University of Hamburg, Germany
Prof. Maya L. Petersen
Professor
University of California at Berkeley
Chair
Els Goetghebeur
Ghent University, Belgium
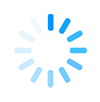